Are you thinking about how to use Generative AI safely?
In the age of data-centric AI, the significance of data access and security cannot be overstated. As different AI and Large Language Model (LLM) systems continue to consume massive amounts of data, the exposure of this sensitive information poses threats to corporate confidentiality and customer privacy alike.
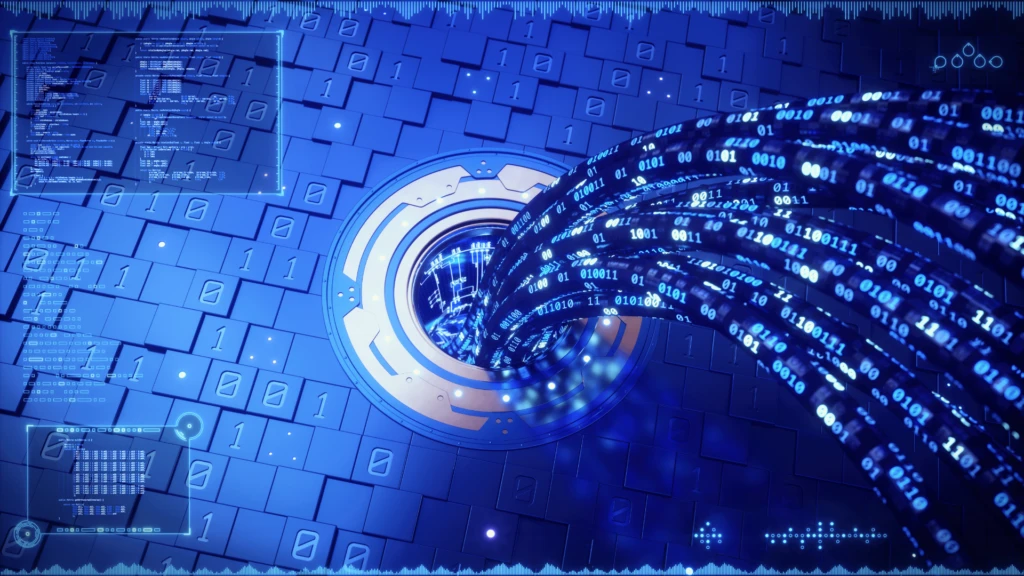
The impact of data breaches on enterprises cannot be underestimated. According to estimates by IBM in 2022, 80% of enterprises report a data breach from AI-generated results. Companies like Apple, Bank of America, Accenture, and Samsung have banned or limited the use of LLMs like ChatGPT internally. The Roomba leak of 2022, where private images of individuals in their homes were exposed, is a cautionary tale. Such incidents can inflict severe damage to a brand’s reputation and erode consumer trust, potentially leading to reduced product adoption.
Challenges Surrounding Data Access and Security in AI
Striking the delicate balance between data gathering and data safety presents several challenges for businesses. One major challenge is the abundance of sensitive information. Although such information can undoubtedly enhance AI models, its utilization is often hindered by data privacy frictions and regulatory constraints.
Additionally, there is the issue of data ownership. Many companies are reluctant to relinquish control of their data to third parties due to various reasons. The consequences of a data leak can be catastrophic, and this reluctance to share data impedes effective collaboration and inhibits progress toward optimal solutions powered by AI.
In addition to the challenges related to sensitive information and data ownership, enterprises face significant hurdles in data access and security due to regulatory constraints and self-imposed policies. These factors play a crucial role in shaping how companies handle data and restrict the use of certain tools, including programs like ChatGPT.
Several government regulations and industry-specific controls have been implemented to protect data privacy and ensure responsible data handling practices. One prominent example is the General Data Protection Regulation (GDPR) implemented by the European Union. Companies that operate within the EU or deal with EU citizens’ data must comply with GDPR requirements, which include obtaining explicit consent for data usage, implementing adequate security measures, and providing individuals with the right to access and erase their data.
Similarly, other regions and countries have enacted their own data protection laws, such as the California Consumer Privacy Act (CCPA) in the United States and the Personal Information Protection and Electronic Documents Act (PIPEDA) in Canada. These regulations impose obligations on businesses to protect individuals’ personal information and give individuals control over how their data is collected and used.
Some companies have also implemented internal policies that restrict or closely monitor the use of AI tools like chatbots for data generation or customer interactions. This cautious approach ensures that the generated content aligns with the organization’s brand values, regulatory requirements, and ethical standards.
In the midst of these numerous challenges, ensuring data access and security become complex tasks. Businesses require access to larger data sets to fully leverage the capabilities of data-centric AI, but nearly half of a company’s data may be inaccessible due to its entanglement with sensitive datasets. The risk of unintentional data leaks further exacerbates the situation, as evidenced by a recent Egress survey indicating that 83% of US companies have inadvertently exposed sensitive information.
4 Simple Steps to Effectively Balance Data Access and Security
Fortunately, businesses can work towards achieving the delicate equilibrium between data gathering and data safety, fostering a secure and privacy-conscious environment in the era of data-centric AI. It’s not always easy to know where to begin, which is why we’ve outlined four steps to data access and security success below.
- Establish clear boundaries with data classification
Begin by clearly defining the boundaries regarding the usability of different types of data in specific contexts. Implement data classification to categorize data into groups such as confidential, internal, and public, enabling effective management of access to protected data.
Solutions like Protopia AI’s AI Stained Glass Transform™ offer lightweight alternatives that allow teams to utilize sensitive data for AI without compromising data ownership. Stained Glass is a lightweight solution that enables teams to use sensitive data for AI without giving up data ownership. By leveraging such solutions, organizations can tap into the value of their data without exposing plaintext information while also ensuring strict controls and safeguards for sensitive data.
- Implement access controls
After classifying data based on sensitivity levels, enforce corresponding access controls. Deploy robust authentication mechanisms, including multi-factor authentication, and employ granular access controls to ensure that only authorized individuals can access and handle sensitive data. Ensure people dealing with sensitive data are officially trained and compliant in working with the information. - Conduct regular security audits and monitoring.
Regularly perform security audits and monitoring of AI systems and data infrastructure. Implement intrusion detection systems, conduct log analysis, and establish real-time monitoring to promptly detect and respond to potential security breaches or unauthorized access attempts. - Employ privacy-preserving techniques.
Utilize privacy-preserving techniques like Randomized Re-Representation or Synthetic data to protect sensitive information while enabling AI models to learn and generate insights. These techniques facilitate data analysis and model training without exposing the underlying sensitive information.
Synthetic data can be particularly useful for data augmentation when data scarcity is a concern. However, it is essential to strike a balance between data restriction and enabling high-functioning AI during actual inferencing, as overly restrictive techniques may hinder AI performance.
Make Your Data AI-Ready
Remember, data accessibility is vital, but striking the right balance by protecting data access and security is even more critical. By taking proactive measures, you can ensure that your data is handled with the utmost care, safeguarding the interests of both your customers and your organization.
Take the first step today by getting in touch with an expert or read our white paper to learn Protopia AI technology can answer key questions about your AI journey.