Overcoming Barriers to Data Accessibility for AI
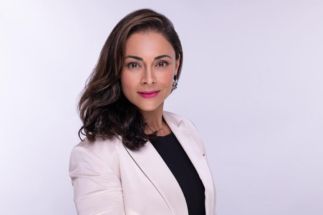
In partnership with Sol Rashidi, former CDAO at Fortune 100 organizations, and best-selling author
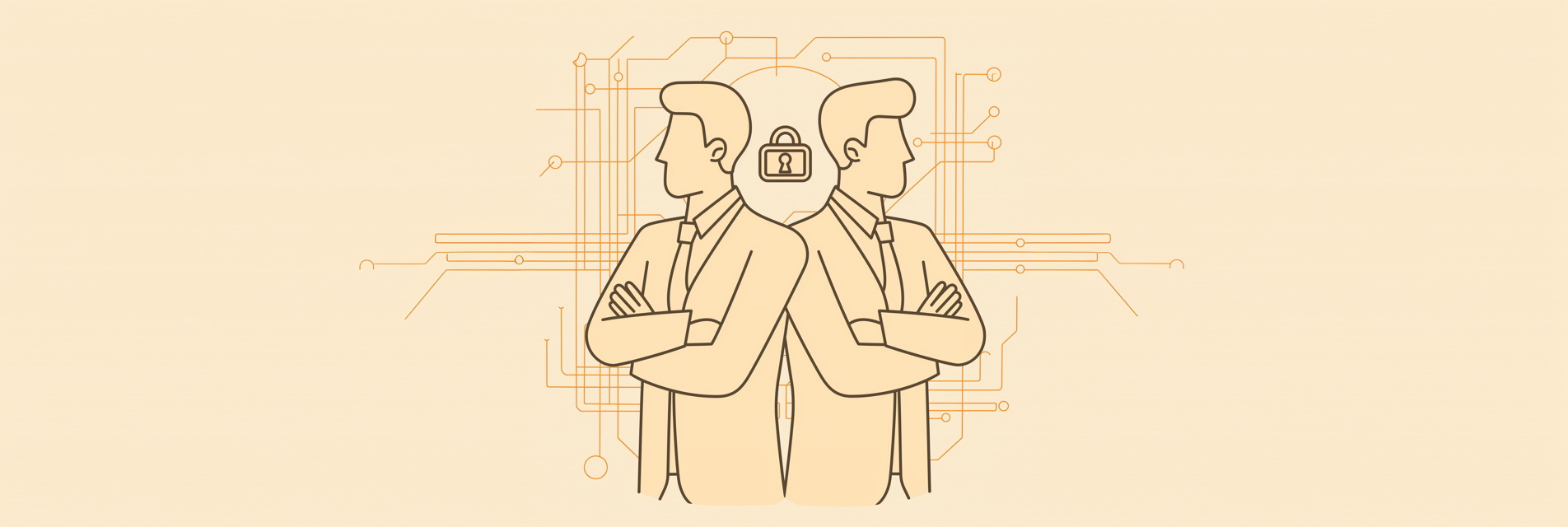
In today’s business landscape, leveraging AI for competitive advantage is no longer optional. Yet, the success of AI initiatives, especially those involving Generative AI, depends crucially on making the right, high-value data available for these projects.
This article is the first in a three-part series that explores how data leaders can leverage their most valuable data to maximize AI efficacy while upholding data confidentiality and privacy.
The series opener tackles the challenge of data accessibility head-on by delving into:
- Navigating Complexities & Competing Agendas How organizational structures, distributed decision-making, and differing incentives across departments (CDOs, CDAOs, CIOs, CISOs) can create roadblocks to accessing and leveraging sensitive data for AI.
- The High Stakes of Sensitive Data Why the most valuable data for AI is often the most tightly controlled, creating a critical tension between innovation and security.
- The Perils of Delay Why waiting for ideal conditions or perfect infrastructure can actually hinder AI adoption and erode your competitive advantage.
- Navigating Organizational Silos How data silos, even within an organization, can stifle collaboration and limit the potential of AI projects.
- The Power of Data Classification Why a collaborative, strategic approach to classifying data is essential for unlocking its value while ensuring appropriate protection.
- Unlocking Data Access How Protopia AI’s Stained Glass Transform safely unlocks access to the most-guarded data while preserving privacy and protection across organizational boundaries and infrastructure deployments.
This article will equip data leaders, including Chief Data Officers (CDOs), Chief Data and Analytics Officers (CDAOs), and Chief Information Officers (CIOs), with actionable strategies to overcome data access challenges and accelerate their AI initiatives securely and responsibly.
The Challenge of Shared Accountability
Gartner’s research reveals that organizations often distribute accountability for AI delivery across multiple leadership roles (Figure 1). While this shared responsibility can be beneficial for holistic strategy development, it often creates hurdles for data and analytics leaders.
High-quality data is the bedrock of impactful business decisions and high-value AI application outcomes. CDOs are tasked with building robust data assets to drive organizational progress, while CIOs are responsible for protecting these assets and determining the infrastructure where data is processed (cloud, on-premises, hybrid environments, etc.). This often creates an innovation barrier where CDOs are limited to using ‘safe’ but sub-optimal subsets of their most valuable data.
Figure 1: The shared accountability for AI initiatives, source: Gartner
The question then becomes: how can data leaders effectively chart a path forward that balances the imperative for innovation with the paramount need for data security? This article explores the relationships, dynamics, and solutions necessary to overcome the daily hurdles associated with leveraging enterprise data for AI initiatives.
Rethinking Data Strategy for Generative AI
The fundamental truth is that ‘AI’ cannot exist without ‘Data,’ and an ‘AI Strategy’ is only as strong as the ‘Data Strategy’ that underpins it. The very data that holds the greatest potential for unlocking insights, driving AI-based capabilities, and creating competitive advantages, is ironically the most tightly controlled and scrutinized.
CDOs and CDAOs face significant challenges in effectively harnessing data, even with well-defined data strategies. While past efforts focused on modernizing technology stacks to handle the ever-growing volume, velocity, and variety of data, the current landscape – marked by the emergence of Generative AI – demands a paradigm shift.
Today’s data leaders are actively seeking ways to expand access to data sets to quickly roll out strategic AI projects. Unlocking access to the most proprietary and guarded data is specially challenging.
As highlighted in recent research by Harvard Business Review (Figure 2), many organizations are still grappling with the development of data strategies specifically tailored for the unique demands of Generative AI.
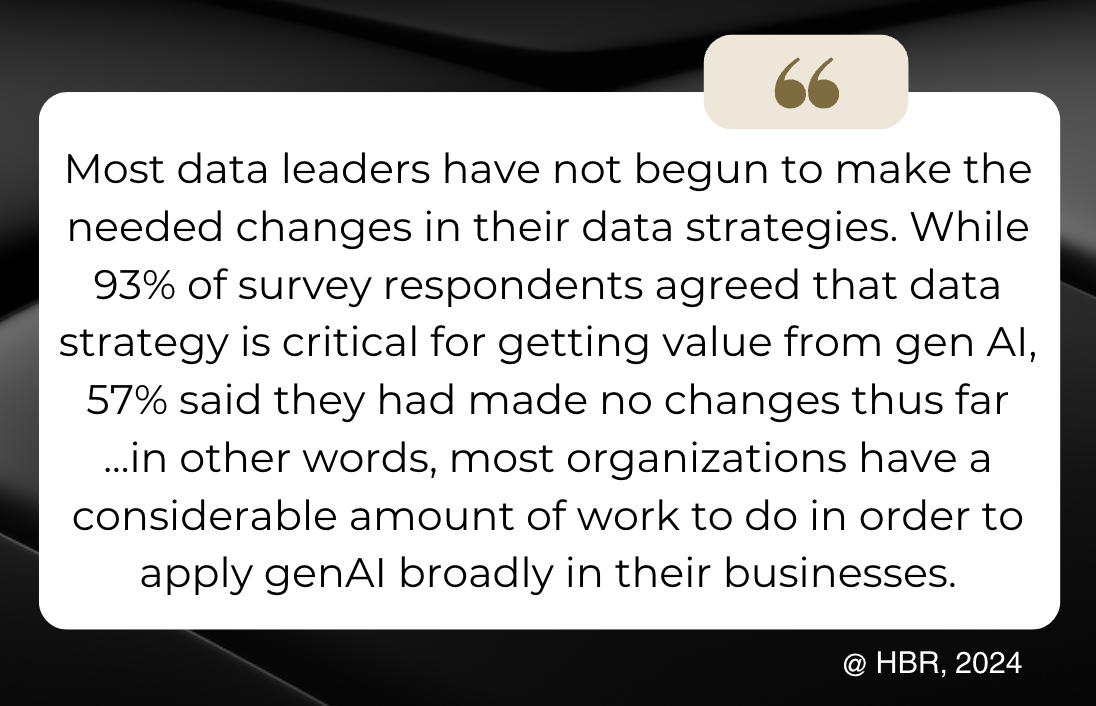
Figure 2: Leaders are yet to action on a new data strategy for GenAI, source: HBR.
The Perils of Evading Sensitive Data
The most sensitive and valuable data is often subject to the most stringent security measures. Consequently, data leaders often avoid exposing such data to AI projects, focusing instead on quick wins or proof-of-concepts with readily available, less sensitive data. Many CDAOs have adopted a ‘wait-and-see’ approach, delaying initiatives until the CIO’s office builds the necessary secure infrastructure (on-premises infrastructure, dedicated GPUs, etc.).
But waiting isn’t the best option for leaders who think beyond the immediate quarter, or for organizations who wish to maximize the ROI of their AI initiatives. This is due to a few reasons:
- GPU shortages: The current surge in AI application development has led to critical GPU shortages, resulting in soaring prices and growing backorders. While procuring GPUs or even getting dedicated GPUs in the cloud can provide some relief, these often prove inadequate. The widespread demand means even hyperscalers can’t always offer enough dedicated compute availability, leading to costly, long-term (often multi-year) reservation requirements.
- Conflicting security stance: A Glean survey revealed conflicting perspectives among CIOs – While 51% believe slowing down AI initiatives is preferable to risking negative consequences, 34% disagree with delaying Generative AI projects given the relentless pace of innovation.
- Competitive disadvantages: Organizations that have become adept at harnessing the power of AI and successfully integrating it into their business processes and workflows have developed this capability through rigorous effort across multiple AI projects. Firms that delay strategic AI projects risk losing their competitive edge as AI adoption grows rapidly.
Consider the following scenarios with sensitive data
- Internal Financial Data Revenue documents, internal financial statements, sensitive business plans etc can be used with AI to identify strategic business opportunities, but must be protected against breaches or exposure.
- Customer Data Information on contact details, purchasing history, preferences, and more can empower personalized campaigns, yet requires careful handling to maintain customer trust and privacy.
- Intellectual Property (IP) Data on patents, proprietary methodologies, etc can be used with AI to conceptualize new innovations and products but be protected to safeguard competitive advantage.
- Legal Documents Contracts, agreements, and other legal communications can streamline procurement, legal, and other functions, but their confidential nature demands strict access controls.
In distributed AI environments, data flows through the hands of many internal and external actors—developers, vendors, users, and ecosystem partners. Ensuring privacy and protection of data within the organization is no longer sufficient; it must persist across the entire supply chain.
This complexity often makes CIOs hesitant to grant AI systems access to potentially sensitive data, raising a crucial question: is it fair to task CIOs and CISOs with sole authorization over what constitutes ‘sensitive’ data when they are often incentivized to label all data as sensitive?
This conundrum inevitably leads to:
- Project Drag and Lag: Increased costs, extended timelines, and mounting frustrations due to protracted discussions, negotiations, and alignment processes.
- Analysis Paralysis: Excessive risk aversion and the potential to miss valuable opportunities due to overemphasizing potential risks over potential rewards.
Prudent CDAOs must explore alternative approaches that facilitate secure data access and accelerate AI adoption, or risk becoming entangled in a blame game when AI rollouts stall.
Data Classification: A Collaborative Approach to Data Stewardship
To overcome these challenges, industry best practices advocate for a collaborative approach to data categorization and classification, engaging both CDOs and CIOs to ensure objectivity and mitigate subjective biases. Data ownership ultimately resides with the business, not with any single executive. Therefore, a collective effort is required to classify corporate data effectively, ensuring its utilization drives business value and supports strategic objectives.
Many organizations fall short in conducting a rigorous assessment of relevant data sets within their data domains, leading to inadequate or subjective tagging that hinders AI application development. Often, organizations subjectively tag data or base tagging on a perceived ‘risk’ associated with the data. When done rigorously, such tagging creates conflicting interpretations and leaves gaps in the protection of your data. Which is why mature data organizations conduct formal, collaborative classifications jointly across CIOs, CISOs, and other technology leaders, typically under the stewardship of the CDO/CDAO office.
This classification has important implications not just for governance and control, but also for the compute/infrastructure used to run AI applications. Let’s examine common classifications (Figure 3):
Figure 3 – How Classification of Enterprise Data Assets Affects Infrastructure Decisions
- Restricted Data At the highest echelon lies restricted data, encompassing proprietary source code, product specifications, trade secrets, research/designs, and financial records. Such data underpins the company’s competitive advantage and existence. These assets often require case-by-case approval for access and utilization.
While this type of data holds the highest potential for driving business value through AI, it is often kept on-premises or in highly secure cloud data platforms, with stringent access controls and robust security measures in place. Organizations also tend to limit the deployment of AI applications utilizing this data to on-premise environments, contending with the growing challenges of procuring and managing the necessary GPUs and infrastructure. Working with such constraints can impede time-to-value of AI projects operating on this sensitive data.
- Confidential Data Next in the hierarchy is confidential data, encompassing organizational documents, revenue details, product designs, and strategic plans. Access to this data is restricted to a select group of individuals for very specific use cases and permissions.
This type of data carries high value for organizational AI initiatives, as it can be used to unlock AI-driven strategic endeavors. However, due to the sensitivity of the data, the corresponding AI applications are often run on dedicated GPUs/compute resources. Dedicated instances incur higher costs, lower scalability due to caps on available resources, and increased maintenance overhead compared to shared compute infrastructure. Waiting for dedicated compute could potentially introduce long delays and considerably slow down the time to value of AI projects.
- Internal Data Forming the third tier of valuable assets is internal data, such as internal documents, scientific research data, geographic information, market research findings, etc, which include information used by internal employees but not intended for public consumption. This class of data has lower sensitivity and can be leveraged more freely within the organization for AI initiatives.
Organizations often run AI applications that consume this tier of data on shared compute infrastructure, balancing lower security requirements with the cost and scalability advantages of a shared environment.
- Public Data Finally, public data, such as news articles, open-source software, public datasets, and public domain multimedia, resides on the internet and is freely accessible to anyone. This non-sensitive information is what most organizations are comfortable using AI applications with today. However, since this data is available to everyone, it is least likely to create competitive advantage.
Although it can be used to create some organizational efficiencies, being restricted to using this class of data with AI is unlikely to generate the return on investments in AI that enterprise leaders will need to demonstrate.
It is therefore reasonable to assume that organizations stand to improve their time to value with AI projects and improve the scalability of their AI systems through solutions, like Protopia AI, that unlock optionality to run their AI workloads on distributed, multi-tenant environments as well as on dedicated GPUs while ensuring privacy.
Additionally, regulatory pressures often lead CIOs to establish further safeguards. This includes data such as personal, financial or healthcare information of personnel and customers, which is subject to privacy regulations like GDPR and CCPA. A breach of such data could result in criminal or civil penalties, identity theft and financial losses. CIOs often implement strict policies, governance and controls on such data for compliance.
This granular classification provides a clear framework for understanding data sensitivity and its potential applications. It is important to note that data sensitivity is not a one-size-fits-all concept; what is considered confidential in manufacturing might be entirely different in healthcare or financial services.
Approaches to Securing Data for AI
Once classified, CDOs and CDAOs must champion the following four (4) deployment tactics:
- Authorization & Authentication: Robust authorization and authentication protocols are paramount for safeguarding sensitive information. Authorization ensures access is granted based on predefined rules and permissions (role, function, use case, etc.), while authentication verifies user identities before granting access. Implementing both in tandem, in collaboration with CIOs and CISOs, ensures that only authorized personnel can access and utilize data within predefined boundaries, protecting the organization’s reputation and bottom line. This endeavor, depending on the size and complexity of the organization, may take up to 14 months due to the need for multi-stakeholder alignment.
- Data Governance for Data Access: Data governance is essential for tracking and monitoring data usage post-classification and authentication. This involves developing comprehensive policies and rules for data access, use, and sharing within the organization. Regular reporting outlining usage patterns, user roles, use cases, accessed data sets, and source/destination targets provide valuable insights into data activity. CIOs, CDOs, and CDAOs should review these reports bi-weekly (at a minimum) to ensure policy adherence and adapt to evolving circumstances.
- Stochastic representations, created by Protopia’s proprietary Stained Glass Transform (SGT) technology, are a powerful approach to unlock your most valuable, private data and expand AI innovation. SGT converts the data that organizations absolutely do not wish exposed on the infrastructure running their AI into randomized representations. These are useless for human interpretation but retain the full utility of the underlying data for AI systems. SGT seamlessly integrates with your existing AI and data protection systems. Our blog, ‘Expanding AI Innovation Securely‘, goes deeper into data classifications and how these transformations ensure utility and protection in the modern AI architecture.
- Private deployments for highly confidential AI workloads: While the cloud offers scalability and cost-efficiency for AI workloads, certain situations demand an even greater emphasis on data security and control. This is particularly true for highly regulated industries or when dealing with extremely sensitive data where on-premises or VPC-based deployments might be the only viable option. However, even within these highly controlled environments, data silos often persist between internal departments, hindering collaboration and limiting the potential of AI initiatives.
Protopia AI’s Stained Glass transformations provide a critical solution for maximizing data security and unlocking collaboration, regardless of deployment strategy. By transforming sensitive data into secure representations, Stained Glass enables:- Secure Data Utilization Across Silos: Departments can confidently share and access valuable data for AI without exposing the raw information, breaking down internal data silos and fostering collaboration.
- Flexibility in Deployment: Whether organizations choose on-premises, VPC, or hybrid deployment models, Stained Glass seamlessly integrates to enhance data security and control without limiting deployment options.
- Enhanced Data Protection for All AI Workloads: Stained Glass isn’t limited to model training or fine-tuning; it protects data throughout the AI lifecycle, including inference, expanding the scope of secure data utilization.
While private deployments offer unparalleled control for specific use cases, Stained Glass emerges as a foundational technology for enabling secure and collaborative data utilization across all deployment strategies, maximizing the value and impact of AI initiatives.
- Secure Data Utilization Across Silos: Departments can confidently share and access valuable data for AI without exposing the raw information, breaking down internal data silos and fostering collaboration.
Trade-offs of Fine-tuning and Training AI with Sensitive Data
Fine-tuning or training AI models with sensitive data presents a nuanced challenge, requiring careful consideration of both risks and rewards. While utilizing real, rich, contextual data can significantly expand potential use cases and enhance model accuracy and reliability, mishandling such data can have severe consequences. Additionally, by enabling the use of smaller, more specialized AI models that are fine-tuned for specific tasks, organizations can achieve substantial cost savings, increase operational efficiency, and potentially reduce their risk profile, all while maintaining a high level of accuracy and performance.
CDOs/CDAOs, in collaboration with CIOs and CISOs, must ensure that data used for AI adheres to the highest standards of privacy and security. This may involve implementing PETs like Protopia AI’s Stained Glass-generated stochastic representations, data masking, differential privacy techniques, or utilizing synthetic data as proxies for real datasets during fine-tuning and training.
The decision to leverage valuable, sensitive data for AI necessitates a thorough risk-benefit analysis. CDAOs should consider not only the immediate benefits of enhanced AI capabilities but also the potential long-term implications of data breaches. Conducting comprehensive risk assessments and establishing robust incident response plans are crucial for mitigating potential risks. While striving for absolute data protection 24/7 is unrealistic, proactive preparation and mitigation plans can effectively address the rare occurrence of breaches.
Cultivating a Culture of Data-Driven Success
In this dynamic and ever-evolving field of data and AI, continuous learning and adaptation are essential for success. CDOs and CDAOs should actively engage with professional communities, startups, and researchers at the forefront of AI data security technologies to remain abreast of the latest advancements and innovative approaches.
Fostering a culture of innovation within the data team is equally important. Encouraging safe experimentation within data governance boundaries can lead to breakthroughs in utilizing sensitive data effectively and securely.
Data, in today’s business environment, is more than just a resource; it is the foundation of innovation and adaptability. As CDOs and CDAOs navigate the intricate balance between leveraging data for AI and maintaining stringent security standards, their focus must remain on maximizing value while minimizing vulnerability. By advocating for sophisticated, secure data management strategies and cultivating strong collaborations across the C-suite, CDOs and CDAOs can unlock the full potential of their data assets, propelling their companies forward in the age of AI.
Looking Ahead
This was the first installment of our three-part series. Here’s what to expect in the coming articles:
Part 2: Navigating the Labyrinth: Data Risks and Classification
A deep dive into data risks when creating AI, exploring popular risk frameworks, and how to progress to risk mitigation after classifying your data assets.
Part 3: Architecting for Trust: Building Systems for Privacy-Preserving AI
Exploring the architectural patterns for building secure AI systems, the enclave vs. hybrid environment debate, and how Protopia expands secure access to valuable data while minimizing risks.
Subscribe to our blog to receive the content in your inbox.
To learn more about how Protopia can help to effectively lead data initiatives and enhance the security of your data flows for AI, please contact our team of experts.
About the Contributor
We are grateful to Sol Rashidi, former CDO, CAO, and CAIO of Fortune 100 companies, such as Estee Lauder, Merck, Sony Music, and Royal Caribbean. Sol holds eight patents and has received numerous accolades, including ‘Top 100 AI People’ 2023, ‘Top 75 Innovators of 2023,’ and ‘Forbes AI Maverick of the 21st Century’ 2022. She has also been recognized as one of the ‘Top 10 Global Women in AI & Data’ 2023, ’50 Most Powerful Women in Tech’ 2022, and appeared on the ‘Global 100 Power List’ from 2021 to 2023 and ‘Top 100 Women in Business’ 2022.